DATA ANALYSIS - 4 MAJOR TYPES
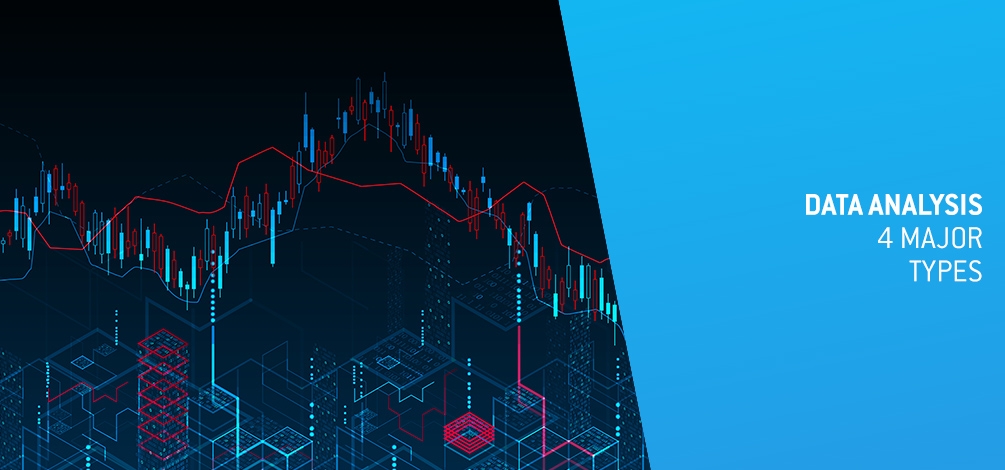
Data analysis can have different approaches, depending on the types of results sought. The complexity of the analysis tends to increase, almost proportionally, along with the value added by the type of analysis.
The modernization of industries and the growing presence and reliance on information technologies has amplified the amount of data obtained. It is raw information, with a lot of value for organizations. These blocks of data contain all kinds of information, which need to be “polished” so that it can be transformed into an asset with added value. This handling of raw data is called data analysis. Data analysis promotes the understanding of information and highlights organizations to offer greater competitiveness to the market. The added value of this side of business impacts the several layers that shape up the organizations:
- Improve efficiency;
- Understand the market;
- Cost reduction;
- Better and fast decision-making;
- New products/services;
- Greater competitiveness;
- Knowledge of the sector;
The first step for organizations is to understand the type of data analysis that best suits their business model and that can boost ROI, as quickly as possible.
Types of data analysis
Data analysis can have different approaches, depending on the types of results sought. The complexity of the analysis tends to increase, almost proportionally, along with the value added by the type of analysis. In this chapter, we choose to consider the 4 types of analysis with the greatest distinction in the market.
Figure 1 - Comparative chart between value and complexity of each analysis
Descriptive
Descriptive analyzes represent a more superficial and less complex layer. This analysis can resume the raw data, converting it to a format that is easy for users to interpret. Descriptive analysis allows to notice patterns and identify problems, extracting the best interpretation possible, to help define strategies that can improve these points. This type of analysis is, above all, associated with the processing of data from the past and in real time, such as KPI's assessment and sales analysis. However, it is one of the types of data analysis most used in the organizations.
Diagnostic
The diagnostic analysis focuses on the reasons implicit in the results of the descriptive analysis. As the name suggests, this analysis tries to find out the why? of the results obtained in the previous analysis. The data processing, according to this type of analysis, aims to find out trends and correlations in information. The increased degree of complexity of the diagnostic analysis, implies the use of data mining techniques to, in a deeper perspective, find a context for the detected problem.
Predictive
Predictive data analysis supposes what is likely to happen in the future. In this type of research, trends are driven from data from the past, used to form logical predictions about the future of a particular context in the organization. Predictive analysis involves advanced tools and reliable technologies so that the result obtained be reliable. Technologically speaking, machine learning and neural networks are probably the main drivers of this type of analysis. So that predictive data analysis models can be effective, it is essential to use accurate data, so that out-of-context forecasts do not occur.
Prescriptive
Prescriptive analysis is the most advanced type of analysis, a set of all previous analyzes, which takes advantage of the results, to calculate actions to avoid future problems. It is also recognized for the complexity of the algorithms needed, to implement analysis suited to the business context. Artificial Intelligence is framed in this type of analysis, consuming a lot of data to learn and make decisions based on the data. This is a very expensive analysis, so it is still used by a small number of organizations.
Streamlined analysis
Tekon IoT Platform, as a solution oriented to data storage and processing, has a section entirely dedicated to data analysis, which simplifies this approach and allows the user to observe and decode behaviours of his application. The detailed information, existing in the collected data, may not be tangible when in the moment of viewing data in real time. Attention to detail tends to be reduced, focusing attention on normal operating patterns and more abrupt offsets.
Figure 2 - "Data analysis" page from Tekon IoT Platform
Across this section, users stipulate the purpose of their analysis, through the configuration of relational parameters such as variables, aggregation type, data aggregation interval and analysis granularity. The combination of all these parameters, results in a chart drawn up automatically, where the interaction actions promote the behavioural understanding of the represented data. The options of data export continue to guarantee the further observation of the analyzes carried out on the Tekon IoT Platform.
Curiosity
In an age where data are constantly used to find patterns and trends, we leave you with a small example of how they can behave similarly and, at the same time, not be interconnected in any way. The Tylervigen website shows us some relationships, like the one shown in the following picture.